|
Monday, 24 April 2017, 11:43 HKT/SGT | |
| | | | Source: Fujitsu Ltd | |
|
|
KAWASAKI, Japan, Apr 24, 2017 - (JCN Newswire) - Fujitsu Laboratories Ltd. today announced the development of circuit technology to improve the energy efficiency of hardware used for deep learning, without changing network structures or training algorithms, by reducing the bit width of the data used in deep learning processing.
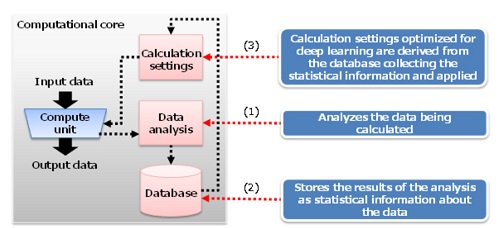 | Figure 1: Improving calculation accuracy in the computational core |
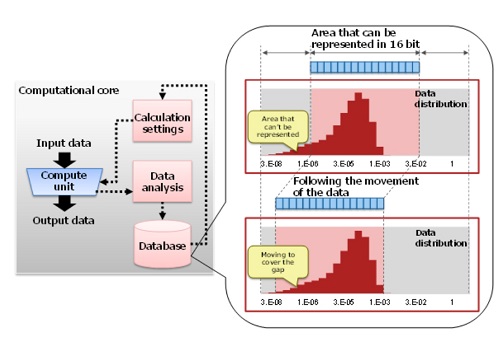 | Figure 2: Optimizing calculation settings using statistical information
|
In the deep learning process, it is necessary to do massive calculations based on training data, but the upper limit to processing performance is determined by the volume of electricity that can be used by the servers and other hardware that carry out the learning processing, so increasing performance per watt has become an issue in accelerating deep learning processing. Now, Fujitsu Laboratories has developed a computational algorithm-driven circuit technology that has a unique numerical representation that reduces data bit width used in computations, and based on the characteristics of deep learning's training computations, automatically controls the location of the decimal point position according to the statistical information of the distribution in order to preserve the computational accuracy sufficiently necessary for deep learning. In this way, in the learning process, the compute unit's bit width and the bit width of the memory that records learning results can be reduced, and energy efficiency can be enhanced. In a simulation of deep learning hardware incorporating this technology, Fujitsu Laboratories confirmed that it significantly improved energy efficiency, by about four times that of a 32-bit compute unit, in an example of deep learning using LeNet(1). With this technology, it has now become possible to expand the range of applicability for advanced AI using deep learning processing to a variety of locations, including servers in the cloud and edge servers. Fujitsu Laboratories intends to commercialize this technology as part of Human Centric AI Zinrai, Fujitsu Limited's AI technology. Details of this technology are scheduled to be announced at xSIG 2017 (The 1st. cross-disciplinary Workshop on Computing Systems, Infrastructures, and Programming), being held at the Toranomon Hills Forum (Minato-ku, Tokyo) April 24-26. Development Background
A topic of discussion in recent years has been that with the spread of IoT, the number of devices connected to the network is increasing dramatically, and that by 2020, tens of billions of devices will be connected. The result will be the generation of staggering amounts of data, although in many cases it will have no meaning as is. The value of this data will have to be extracted using machine learning methods, such as deep learning, which is expected to create new insights. With the increase in training data from IoT and the expanding scale of deep neural networks, the performance requirements for servers used for deep learning have only been increasing. In addition, in order to reduce the communications volume necessary for transmitting data and the storage volume necessary for recording it, there is a growing need to handle deep learning not only in the cloud, but also at the edge, close to the places where the data is generated. Because servers used for deep learning, both in the cloud and at the edge, have power limits, forecasters expect that it will become difficult to increase performance simply by increasing scale, making technologies that raise energy efficiency necessary. Issues
The hardware used in ordinary deep learning uses a data format called 32-bit floating-point representation for processing calculations. By reducing the bit width of data used in calculations to 16 bits or less, or using hardware that uses integer operations for calculations, the volume of calculations can be reduced, increasing energy efficiency. At the same time, however, this can lead to a lack of the accuracy necessary for these calculations, making deep learning impossible or degrading the recognition capability of the neural network. About the Newly Developed Technology
Fujitsu Laboratories has now developed circuit technology, based on integer operations, that improves energy efficiency by reducing the data bit width of the compute units and the memory that records training results of deep learning, using a unique numerical representation to reduce bit width. The computational algorithm controls the location of the decimal point in order to preserve computational accuracy while analyzing the distribution of the data for each layer of a deep neural network. Both are specialized for the deep learning process. In the computational cores of the hardware used for deep learning using this technology, there is a block devoted to analyzing the data being calculated (1), a database that stores the distribution of the analyzed data (2), and a block that preserves the calculation settings (3) (Figure 1). In the data analysis block, the data output of the compute unit is analyzed in real time during the training of neural networks, and that analysis is stored in the database as statistical information showing the distribution of the data. That distribution is used to configure compute unit settings, so that it can preserve sufficient computational accuracy in order to improve training accuracy (Figure 2). http://www.acnnewswire.com/topimg/Low_FujitsuCircuitTechFig1.jpg Figure 1: Improving calculation accuracy in the computational core
http://www.acnnewswire.com/topimg/Low_FujitsuCircuitTechFig2.jpg Figure 2: Optimizing calculation settings using statistical information Effects
Fujitsu Laboratories has confirmed that, applying this newly developed technology, a system using LeNet and the MNIST(2) dataset as a learning target was able to achieve a recognition rate of 98.31% with 8 bits and a recognition rate of 98.89% with 16 bits, almost identical compared with a recognition rate of 98.90% using 32-bit floating-point operations. This newly developed circuit technology improves energy efficiency in two ways. One is that power can be reduced by executing operations that were done in floating point as integer calculations, instead(3). The second way is by reducing data bit width from 32 bit to 16 bit to cut the volume of data being handled in half, meaning that the power consumption of the compute unit and memory can be reduced by about 50%. Moreover, by reducing it to 8 bit, the power consumption of the compute unit and memory can be reduced by about 75%. In this way, by improving the energy efficiency of the hardware used for deep learning, Fujitsu Laboratories has made it possible to shift deep learning processing, which requires large volumes of training data, from cloud servers to edge servers close to where the data is generated. Future Plans
Fujitsu Laboratories plans to work with customers on AI applications, with the goal of commercializing this technology as part of Human Centric AI Zinrai, Fujitsu Limited's AI technology, in fiscal 2018. It will also continue to develop circuit technology in order to further reduce the data volumes used in deep learning. (1) LeNet A convolutional neural network used for recognizing handwritten numbers. (2) MNIST A training data set of 28x28-pixel handwritten numbers for recognition. (3) There is data that the total power used for multipliers and adders for 32-bit integer data is about 3.2 pico joules (pJ), while that used for 32-bit floating-point data is about 4.6 pJ M. Horowitz, "1.1 Computing's energy problem (and what we can do about it)," 2014 IEEE International Solid-State Circuits Conference Digest of Technical Papers (ISSCC), San Francisco, CA, 2014, pp. 10-14.
About Fujitsu Laboratories
Founded in 1968 as a wholly owned subsidiary of Fujitsu Limited, Fujitsu Laboratories Ltd. is one of the premier research centers in the world. With a global network of laboratories in Japan, China, the United States and Europe, the organization conducts a wide range of basic and applied research in the areas of Next-generation Services, Computer Servers, Networks, Electronic Devices and Advanced Materials. For more information, please see: http://www.fujitsu.com/jp/group/labs/en/.
Contact:
Fujitsu Laboratories Ltd.
Computer Systems Laboratory
E-mail: ngcs_ai_press_arc@ml.labs.fujitsu.com
Fujitsu Limited
Public and Investor Relations
Tel: +81-3-3215-5259
URL: www.fujitsu.com/global/news/contacts/
Topic: Press release summary
Source: Fujitsu Ltd
Sectors: Electronics
http://www.acnnewswire.com
From the Asia Corporate News Network
Copyright © 2025 ACN Newswire. All rights reserved. A division of Asia Corporate News Network.
|
|
|
|
|
|
Fujitsu Ltd |
Mar 12, 2025 12:53 HKT/SGT |
MOL and Fujitsu leverage AI for efficient crew replacement planning |
Mar 3, 2025 17:23 HKT/SGT |
Rakuten Mobile Partners with Fujitsu to Accelerate 5G Network Expansion |
Mar 3, 2025 16:58 HKT/SGT |
Fujitsu unveils 1FINITY 800G ZR/ZR+ coherent pluggable transceivers |
Feb 28, 2025 15:57 HKT/SGT |
Fujitsu's Oyama plant achieves top CSR score for sustainability in global telecom audit |
Feb 25, 2025 10:24 HKT/SGT |
Fujitsu, ANA X, Toshiba Data, and Kawasaki City launch Japan's first pilot program to quantify citizen CO2 reduction |
Feb 13, 2025 09:01 HKT/SGT |
Fujitsu to offer Fujitsu Cloud Service Generative AI Platform for secure and flexible enterprise data management |
Feb 12, 2025 10:37 HKT/SGT |
Fujitsu and Yokohama National University achieve world's first real-time prediction of tornadoes associated with typhoons using supercomputer Fugaku |
Feb 5, 2025 09:25 HKT/SGT |
Fujitsu to highlight AI-powered network technologies at MWC Barcelona 2025 |
Feb 4, 2025 10:39 HKT/SGT |
Fujitsu launches gen AI software analysis and visualization service to support optimal modernization planning |
Feb 3, 2025 11:05 HKT/SGT |
Fujitsu and Tokai National Higher Education and Research System leverage explainable AI to enhance space weather prediction in collaboration with JAXA |
More news >> |
 |
|
 |
|