|
Wednesday, 19 September 2018, 16:25 HKT/SGT | |
| | | | Source: Fujitsu Ltd | |
|
|
TOKYO, Sept 19, 2018 - (JCN Newswire) - Fujitsu Laboratories Ltd. today announced the development of "Wide Learning," a machine learning technology capable of accurate judgements even when operators cannot obtain the volume of data necessary for training. AI is now often used to leverage data in a variety of fields, but the accuracy of AI may be impacted in cases where the volume of data to be analyzed is small or imbalanced. Fujitsu's Wide Learning technology enables judgements to be reached more accurately than was previously possible, and learning is achieved uniformly, no matter which hypothesis is examined, even when the data is imbalanced. It achieves this by first extracting hypotheses with a high degree of importance, having made a large set of hypotheses formed by all of the combinations of data items, and then by controlling for the degree of impact of each respective hypothesis based on the overlapping relationships of the hypotheses. Moreover, because the hypotheses are recorded as logical expressions, humans can also understand the reasoning behind a judgement. Fujitsu's new Wide Learning technology allows for the use of AI even in areas such as healthcare and marketing, where the data needed to make judgements is scarce, supporting operations and promoting the automation of work processes using AI.
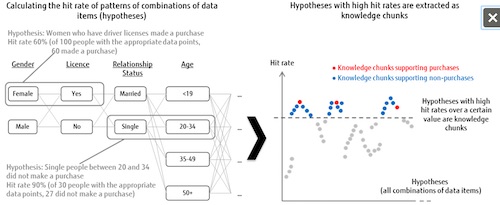 | Figure 1: Hypothesis listing and knowledge chunk extraction |
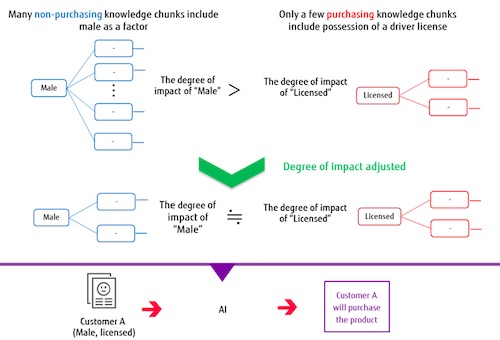 | Figure 2: When making a classification model, the knowledge chunks impact adjustment |
Development Background
In recent years, AI technology has begun to be used in a variety of fields, including healthcare, marketing, and finance. Expectations are rising for the use of AI decision-making in support of operations and automating tasks in these areas. One challenge that remains to realizing the potential of these technologies, however, is that the data may be imbalanced. Specifically, depending on the industry it can be difficult to obtain sufficient data for training AI on the targets on which it is to make judgements. This, in effect, leaves many of these technologies unable to produce results with sufficient accuracy for practical use. Furthermore, a major reason why AI deployment lacks progress is that even when an AI provides sufficiently accurate recognition or classification performance, experts and even the developers themselves often cannot explain why the AI produced a certain answer, and if they cannot fulfill their responsibility to explain the results to the front lines of industry then AI cannot be deployed.
Issues
AI technologies based on deep learning conventionally make highly accurate judgements by being trained on large volumes of data, including ample target data to be judged. In real world scenarios, however, there are many cases in which the data is insufficient, with extremely little target data. In these cases, when faced with unknown data, it becomes difficult for AI technology to deliver highly accurate judgements. Moreover, the machine learning model for existing AI based on deep learning is a black box model that cannot explain the reasons behind the judgements the AI makes, creating a problem with transparency. As such, moving forward it will be necessary to develop new AI technology that realizes highly accurate judgements from imbalanced data, and that is also transparent in order to solve various issues in society.
About the Newly Developed Technology
Bearing these challenges in mind, Fujitsu Laboratories has now developed Wide Learning, a machine learning technology capable of making highly accurate judgements even in cases where the data is imbalanced. The features of Wide Learning technology include the following two points.
1. Creates combinations of data items to extract large volumes of hypotheses
This technology treats all combination patterns of data items as hypotheses, and then determines the degree of importance of each hypothesis based on the hit rate for the label category. For example, when analyzing trends in who purchases certain products, the system combines all sorts of patterns from the data items for those who did or did not make purchases (the category label), such as single women between 20-34 who have driver licenses, and then analyzes how many hits it gets in the data of those who actually made purchases when these combination patterns are taken as hypotheses. The hypotheses that achieve a hit rate above a certain level are defined as important hypotheses, called "knowledge chunks". This means that even when target data is insufficient, the system can extract all hypotheses worth looking into, which may also contribute to the discovery of previously unconsidered explanations.
http://www.acnnewswire.com/topimg/Low_FujitsuNewAITechnology.jpg Figure 1: Hypothesis listing and knowledge chunk extraction
2. Adjusts the degree of impact of knowledge chunks to build an accurate classification model
The system builds a classification model based on multiple extracted knowledge chunks and on the target label. In this process, if the items making up a knowledge chunk frequently overlap with the items making up other knowledge chunks, the system controls their degree of impact so as to reduce the weight of their influence on the classification model. In this way, the system can train a model capable of accurate classifications even when the target label or the data marked as correct is imbalanced. For example, in a case where men who did not make a purchase make up the vast majority of an item purchase dataset, if the AI is trained without controlling the degree of impact, then the knowledge chunk that includes whether or not a person has a license, independent of gender, will not have much influence on the classification. With this newly developed method, the degree of impact of knowledge chunks including male as a factor is limited due to the overlap of this item, while the impact of the smaller number of knowledge chunks that include whether a person has a license becomes relatively larger in training, building a model that can correctly categorize both men and possession of a license.
http://www.acnnewswire.com/topimg/Low_FujitsuNewAITechnology2.jpg Figure 2: When making a classification model, the knowledge chunks impact adjustment
Effects
Fujitsu Laboratories conducted a trial of this technology, applying it to data in areas such as digital marketing and healthcare. In a test using benchmark data in the marketing and healthcare areas from the UC Irvine Machine Learning Repository(1), this technology improved accuracy by about 10-20% compared to deep learning. It successfully reduced the probability that the system would overlook customers likely to subscribe to a service or patients with a condition by about 20-50%. In the marketing data, of the approximately 5,000 customer data entries used in the test, only about 230 were for purchasing customers, making for an imbalanced set. This technology reduced the number of potential customers excluded from sales promotions from 120, the result of deep learning analysis, to 74. Moreover, as the knowledge chunks that form the basis for this technology have a logical expression format, the ability to explain the reasoning behind a judgement is also useful in implementing this technology in society. Even when it is determined that corrections to a model are necessary, based on results from new data, it is possible to make more appropriate revisions, because users can understand the reasons for results.
Future Plans
Fujitsu Laboratories will continue to apply this technology to tasks that demand the reasoning behind AI judgements, such as in financial transactions and medical diagnoses, and to tasks that handle low frequency phenomena, such as fraud and equipment breakdowns, with the goal of commercializing it as a new machine learning technology supporting Fujitsu Limited's Fujitsu Human Centric AI Zinrai in fiscal 2019. Fujitsu Laboratories will also make effective use of this technology's characteristic capability for explanation, continuing research and development into topics such as improved support for making judgements and decisions in tasks to which it is applied, and into the overall system design, including collaboration with humans.
(1) UC Irvine Machine Learning Repository A world-famous repository that provides numerous datasets for use in evaluating and comparing machine learning.
About Fujitsu Laboratories
Founded in 1968 as a wholly owned subsidiary of Fujitsu Limited, Fujitsu Laboratories Ltd. is one of the premier research centers in the world. With a global network of laboratories in Japan, China, the United States and Europe, the organization conducts a wide range of basic and applied research in the areas of Next-generation Services, Computer Servers, Networks, Electronic Devices and Advanced Materials. For more information, please see: http://www.fujitsu.com/jp/group/labs/en/.
Contact:
Fujitsu Laboratories Ltd.
Artificial Intelligence Laboratory
E-mail: widelearning@ml.labs.fujitsu.com
Fujitsu Limited
Public and Investor Relations
Tel: +81-3-3215-5259
URL: www.fujitsu.com/global/news/contacts/
Topic: Press release summary
Source: Fujitsu Ltd
Sectors: Electronics, Cloud & Enterprise
http://www.acnnewswire.com
From the Asia Corporate News Network
Copyright © 2025 ACN Newswire. All rights reserved. A division of Asia Corporate News Network.
|
|
|
|
|
|
Fujitsu Ltd |
Apr 24, 2025 15:24 HKT/SGT |
Fujitsu launches new company 1FINITY to strengthen network products business
|
Apr 23, 2025 10:55 HKT/SGT |
Fujitsu expands strategic collaboration with Supermicro to offer total generative AI platform |
Apr 22, 2025 10:37 HKT/SGT |
Fujitsu and RIKEN develop world-leading 256-qubit superconducting quantum computer |
Apr 18, 2025 16:41 HKT/SGT |
Fujitsu Kozuchi AI technologies assist AKOS AI in delivering solutions for EU AI compliance |
Apr 17, 2025 09:32 HKT/SGT |
Fujitsu collaboration with Supermicro and Nidec to reduce data center energy consumption |
Apr 16, 2025 10:24 HKT/SGT |
Fujitsu's Japanese language enhanced LLM Takane offering now available on Nutanix Enterprise AI solution |
Apr 15, 2025 11:29 HKT/SGT |
Isuzu and Fujitsu sign partnership agreement to commercialize software defined vehicles |
Apr 11, 2025 10:29 HKT/SGT |
Fujitsu develops employee training platform for Seven-Eleven Japan to accelerate store digitalization |
Apr 11, 2025 08:07 HKT/SGT |
Fujitsu and Headwaters trial on-device generative AI solution to streamline JAL cabin crew workflows |
Apr 9, 2025 09:30 HKT/SGT |
Sonofai revolutionizes frozen tuna inspection with new device incorporating Fujitsu AI tech |
More news >> |
 |
|
 |
|