|
Monday, 16 March 2020, 10:42 HKT/SGT | |
| | | | Source: Fujitsu Ltd | |
|
|
|
New Technology reduces the number of man hours required for creating AI models leveraging Topological Data Analysis (TDA) by a factor of 100, accelerating field deployment |
TOKYO, Mar 16, 2020 - (JCN Newswire) - Fujitsu Limited, Fujitsu Laboratories Ltd., and Inria, the French national research institute for digital science and technology, today announced the development of technology that automatically creates AI models capable of detecting anomalies in time-series data taken from IoT devices and other sources. With the continued progress of AI technology in recent years, there has been greater deployment of AI in a variety of business fields. Despite demand for greater levels of automation, the most common means of creating AI models still involve painstaking, manual work by specialized AI engineers. Moreover, because the process of building new AI models continues to rely on trial and error, it demands significant man-hours, often leading to delays in-field deployment.
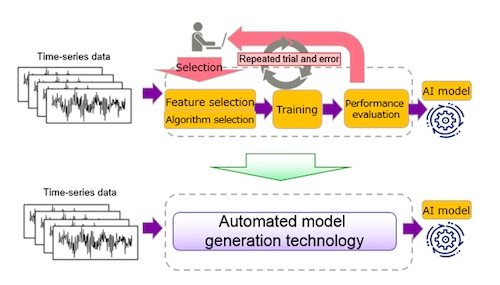 | Figure 1: AI model creation process before and after deploying this new technology |
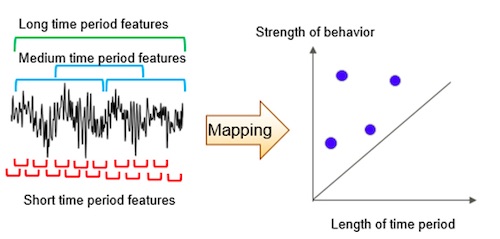 | Figure 2: Mapping the various features of time-series data to a plane |
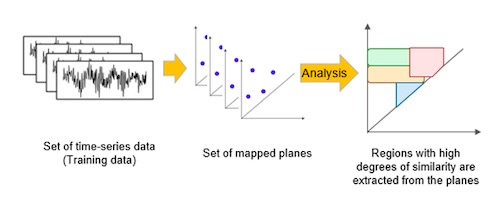 | Figure 3: Generating an AI model using time-series training data |
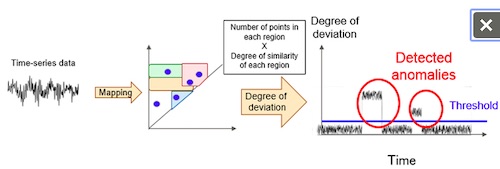 | Figure 4: Evaluating unknown time-series data using the AI model |
A new jointly developed technology to automatically create anomaly-detecting AI Models
Leveraging proprietary time-series data analysis technology developed by Fujitsu Laboratories that utilizes improved topological data analysis (TDA)(1), Fujitsu and Inria project-team DataShape have now developed a new technology to automatically create AI models that can detect anomalies by extracting the necessary information from time-series data. Time-series data, which can include sensor data from IoT devices or biological data, such as heart rates and brain waves, consists of information of a wide range of types with complicated interconnections. This means that time-series data is often subject to severe volatility, making it difficult to discern when meaningful patterns or anomalies occur in the data. This technology enables any software engineer to easily create AI categorization and anomaly detection models for time-series data, while also reducing the man-hours required to one hundredth that of previous methods. This will ultimately help to accelerate the deployment of new AI models in a variety of business fields, allowing even engineers with no specialized training to create anomaly detection models.
Trials demonstrate significant improvement in AI models for real-world use through new technology
Trials were conducted using newly-developed technology for automatically generating anomaly detection models. These successfully demonstrated considerable gains in efficiency that will help accelerate the deployment of AI models to solve real-world problems:
- An AI model for detecting internal damage in bridges When evaluated using vibration data equivalent to 30 years worth of data collected from accelerometers attached to a mock bridge deck plate(2) for experimental use, a model with the same detection performance as the AI model developed by specialist AI engineers over 5 days was created in 10 minutes.
- An AI model for detecting anomalous states, such as drowsiness, in human pulse data This technology took twenty minutes to create a model that had one-tenth the average error of an AI model created over the course of four days by AI specialist engineers using standard methods.
Availability and Future Plans
This newly developed technology has been incorporated into GUDHI, an open-source TDA library developed by Inria, and will be available for users globally for free from March 16. This will not only promote the use of AI in companies, research institutions, and other organizations--it will also enable the creation of AI models for a variety of use cases as feedback from those organizations is reflected in ongoing technology improvements. Fujitsu Laboratories will continue to refine this approach as one of the core technologies supporting its Fujitsu Human Centric AI Zinrai portfolio of solutions. This technology will be presented at the 23rd International Conference on Artificial Intelligence and Statistics (AISTATS 2020), an international conference on machine learning that will be held in Palermo, Italy, from June 3-5.
About the Newly Developed Technology
In the case of time-series data, which includes sensor data and biological data, such as heart rates and brain waves, for example, it is often necessary to extract features of data across a range of different time windows, and there can be a wide range of feature types. If the appropriate combination is not selected, the model will not be able to achieve its target performance, making the automatic generation of AI models extremely difficult. The technology developed by Fujitsu and Inria automatically extracts the necessary information to create anomaly detection models for time-series data. The key features of this technology are as follows:
1. Joint development of an algorithm to extract features from time-series data
Using the proprietary time-series data analysis technology developed by Fujitsu Laboratories, Fujitsu Laboratories and Inria have jointly developed an algorithm to extract features that are important for detecting anomalies in time-series data. Within time-series data, there are some features that appear over short time periods, and some that appear over long time periods, and it is necessary to extract both, as appropriate. Additionally, there are features such as frequency and amplitude in the various sections of segmented data, and many features that cannot be extracted with statistical or frequency analysis methods. With this algorithm, using a Deep Learning technology developed by Fujitsu Laboratories for accurately analyzing time-series data(3), features can be mapped as points on a chart, with the axes defined by the length of the time period and the features of the behavior of the waveform over that period. This enables the user to gain a more comprehensive view of information such as the lengths of time periods and the features of the data's behavior.
2. Extraction of necessary information for anomaly detection from the feature plane
The features of the various segments of time-series training data prepared in advance are each mapped onto these graphs using Fujitsu Laboratories' time-series data analysis technology. These mapped charts are then compared, and the space is divided into regions where ordinary data points co-occur, regions where they do not, and regions where there is no overlapping data. The number of regions and the method of segmentation are then optimized such that the number of feature points within each region is the same, with the strength of the extent of commonality calculated as the degree of similarity, and the regions are then extracted in the order of their degree of similarity. (Figure 3)
Next, for time-series test data, in order to determine if the data is anomalous or not, the features extracted from the input data using the TDA technology are mapped onto a chart, and the number of points which fall into the regions delineated above are counted. By multiplying the number of points that fall within each region with the degree of similarity for that region, and then adding together all the regions, the technology outputs a value representing the degree of deviation, which is then used to determine the deviation of the input data from the standard. (Figure 4)
(1) Topological Data Analysis A method for analyzing data in which data are arrayed in a cluster of points in space, and geometric data is extracted from the cluster. (2) Deck Plate Bridge deck plate, which is the structure that conveys the weight of vehicles passing over bridges to bridge piers (3) Deep learning AI technology developed by Fujitsu Laboratories for accurately analyzing time-series data Proprietary technology from Fujitsu Laboratories that, using TDA, analyzes time-series data with high precision. "Fujitsu Develops New Deep Learning Technology to Analyze Time-Series Data with High Precision," (press release dated February 16, 2016): https://www.fujitsu.com/global/about/resources/news/press-releases/2016/0216-01.html
About Fujitsu Laboratories
Founded in 1968 as a wholly owned subsidiary of Fujitsu Limited, Fujitsu Laboratories Ltd. is one of the premier research centers in the world. With a global network of laboratories in Japan, China, the United States and Europe, the organization conducts a wide range of basic and applied research in the areas of Next-generation Services, Computer Servers, Networks, Electronic Devices and Advanced Materials. For more information, please see: http://www.fujitsu.com/jp/group/labs/en/.
About Inria
Inria is the French national research institute for digital science and technology. World-class research and technological innovation are part of its DNA, with the aim of developing and supporting scientific and entrepreneurial projects that create value for France, within a European perspective. www.inria.fr
Topic: Press release summary
Source: Fujitsu Ltd
Sectors: Artificial Intel [AI], Automation [IoT]
http://www.acnnewswire.com
From the Asia Corporate News Network
Copyright © 2025 ACN Newswire. All rights reserved. A division of Asia Corporate News Network.
|
|
|
|
|
|
Fujitsu Ltd |
Feb 5, 2025 09:25 HKT/SGT |
Fujitsu to highlight AI-powered network technologies at MWC Barcelona 2025 |
Feb 4, 2025 10:39 HKT/SGT |
Fujitsu launches gen AI software analysis and visualization service to support optimal modernization planning |
Feb 3, 2025 11:05 HKT/SGT |
Fujitsu and Tokai National Higher Education and Research System leverage explainable AI to enhance space weather prediction in collaboration with JAXA |
Jan 30, 2025 10:56 HKT/SGT |
Fujitsu and Asepeyo collaborate to modernise the management of occupational benefits and contingencies |
Jan 27, 2025 09:53 HKT/SGT |
Sustainable Shared Transport and Fujitsu launch open platform for joint transportation and delivery in Japan, enhancing logistics efficiency across industries |
Jan 15, 2025 12:13 HKT/SGT |
Fujitsu, Resona Bank and Saitama Resona Bank launch new web service to simplify home-buying process |
Dec 23, 2024 12:30 HKT/SGT |
Fujitsu drives chemical industry logistics DX with participation in joint logistics demonstration |
Dec 16, 2024 09:20 HKT/SGT |
Fujitsu recognized as Leader in IDC MarketScape: Worldwide Digital Workplace Services 2024 Vendor Assessment |
Dec 12, 2024 10:06 HKT/SGT |
Fujitsu develops video analytics AI agent to support safe, secure, and efficient frontline workplaces |
Dec 12, 2024 09:28 HKT/SGT |
Fujitsu develops world's first multi-AI agent security technology to protect against vulnerabilities and new threats |
More news >> |
 |
|
 |
|