|
|
|
|
A model that rapidly searches through large numbers of materials could find sustainable alternatives to existing composites. |
TSUKUBA, Japan, May 25, 2022 - (ACN Newswire) - Researchers from Konica Minolta and the Nara Institute of Science and Technology in Japan have developed a machine learning method to identify sustainable alternatives for composite materials. Their findings were published in the journal Science and Technology of Advanced Materials: Methods.
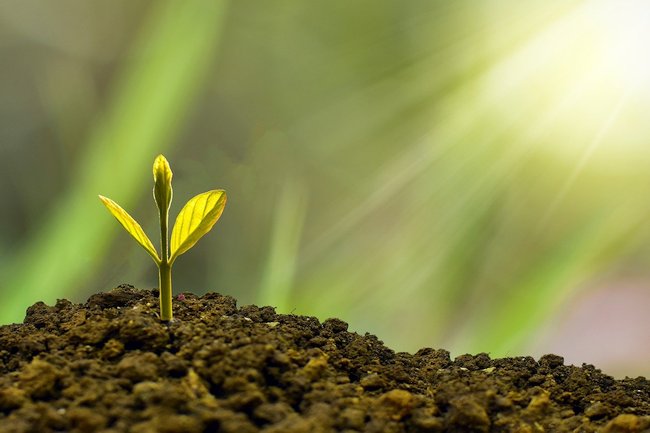 | Researchers are looking for sustainable options, such as recyclable materials or biomass, to substitute the constituent materials in composites which are used in various applications including electrical and information technologies. |
Composite materials are compounds made of two or more constituent materials. Due to the complex nature of the interactions between the different components, their performance can greatly exceed that of single materials. Composite materials, such as fibre-reinforced plastics, are very important for a wide range of industries and applications, including electrical and information technologies.
In recent years, there has been increasing demand for more environmentally sustainable materials that help reduce industrial waste and plastic use. One way to achieve this is to substitute the constituent materials in composites with recyclable materials or biomass. However, this can reduce performance compared to the original material, not only due to the features of the individual constituent materials, such as their physicochemical properties, but also due to the interactions between the constituents.
"Finding a new composite material that achieves the same performance as the original using human experience and intuition alone takes a very long time because you have to evaluate countless materials while also taking into account the interactions between them," explains Michihiro Okuyama, assistant manager at Konica Minolta, Inc.
Machine learning offers a potential solution to this problem. Scientists have proposed several machine learning methods to conduct rapid searches among a large number of materials, based on the relationship between the materials' features and performance. However, in many cases the properties of the constituent materials are unknown, making these types of predictive searches difficult.
To overcome this limitation, the researchers developed a new type of machine learning method for finding alternative materials. A key advantage of the new method is that it can quantitatively evaluate the interactions among the component materials to reveal how much they contribute to the overall performance of the composite. The method then searches for replacement constituents with similar performance to the original material.
The researchers tested their method by searching for alternative constituent materials for a composite consisting of three materials - resin, a filler and an additive. They experimentally evaluated the performance of the substitute materials identified by machine learning and found that they were similar to the original material, proving that the model works.
"In developing alternatives, that make up composite materials, our new machine learning method removes the need to test large numbers of candidates by trial and error, saving both time and money." says Okuyama.
The method could be used to quickly and efficiently identify sustainable substitutes for composite materials, reducing plastic use and encouraging the use of biomass or renewable materials.
Further information Michihiro Okuyama KONICA MINOLTA, INC. Email: michihiro.okuyama@konicaminolta.com
About Science and Technology of Advanced Materials: Methods (STAM Methods)
STAM Methods is an open access sister journal of Science and Technology of Advanced Materials (STAM), and focuses on emergent methods and tools for improving and/or accelerating materials developments, such as methodology, apparatus, instrumentation, modeling, high-through put data collection, materials/process informatics, databases, and programming. https://www.tandfonline.com/STAM-M
Dr. Masanobu Naito STAM Methods Publishing Director Email: NAITO.Masanobu@nims.go.jp
Press release distributed by Asia Research News for Science and Technology of Advanced Materials.
Topic: Press release summary
Source: Science and Technology of Advanced Materials
Sectors: Electronics, Chemicals, Spec.Chem, Science & Nanotech, Artificial Intel [AI]
http://www.acnnewswire.com
From the Asia Corporate News Network
Copyright © 2025 ACN Newswire. All rights reserved. A division of Asia Corporate News Network.
|
|
|
|
|
|
Science and Technology of Advanced Materials |
Jan 28, 2025 08:00 HKT/SGT |
High-brilliance radiation quickly finds the best composition for half-metal alloys |
Dec 3, 2024 23:15 HKT/SGT |
Machine learning used to optimise polymer production |
Oct 25, 2024 23:00 HKT/SGT |
Machine learning can predict the mechanical properties of polymers |
July 30, 2024 20:00 HKT/SGT |
Dual-action therapy shows promise against aggressive oral cancer |
Apr 17, 2024 22:00 HKT/SGT |
A new spin on materials analysis |
Apr 12, 2024 18:00 HKT/SGT |
Kirigami hydrogels rise from cellulose film |
Feb 27, 2024 08:00 HKT/SGT |
Sensing structure without touching |
Nov 21, 2023 07:00 HKT/SGT |
Nano-sized probes reveal how cellular structure responds to pressure |
Nov 17, 2023 10:00 HKT/SGT |
Machine learning techniques improve X-ray materials analysis |
Nov 14, 2023 20:00 HKT/SGT |
A bio-inspired twist on robotic handling |
More news >> |
 |
|
|